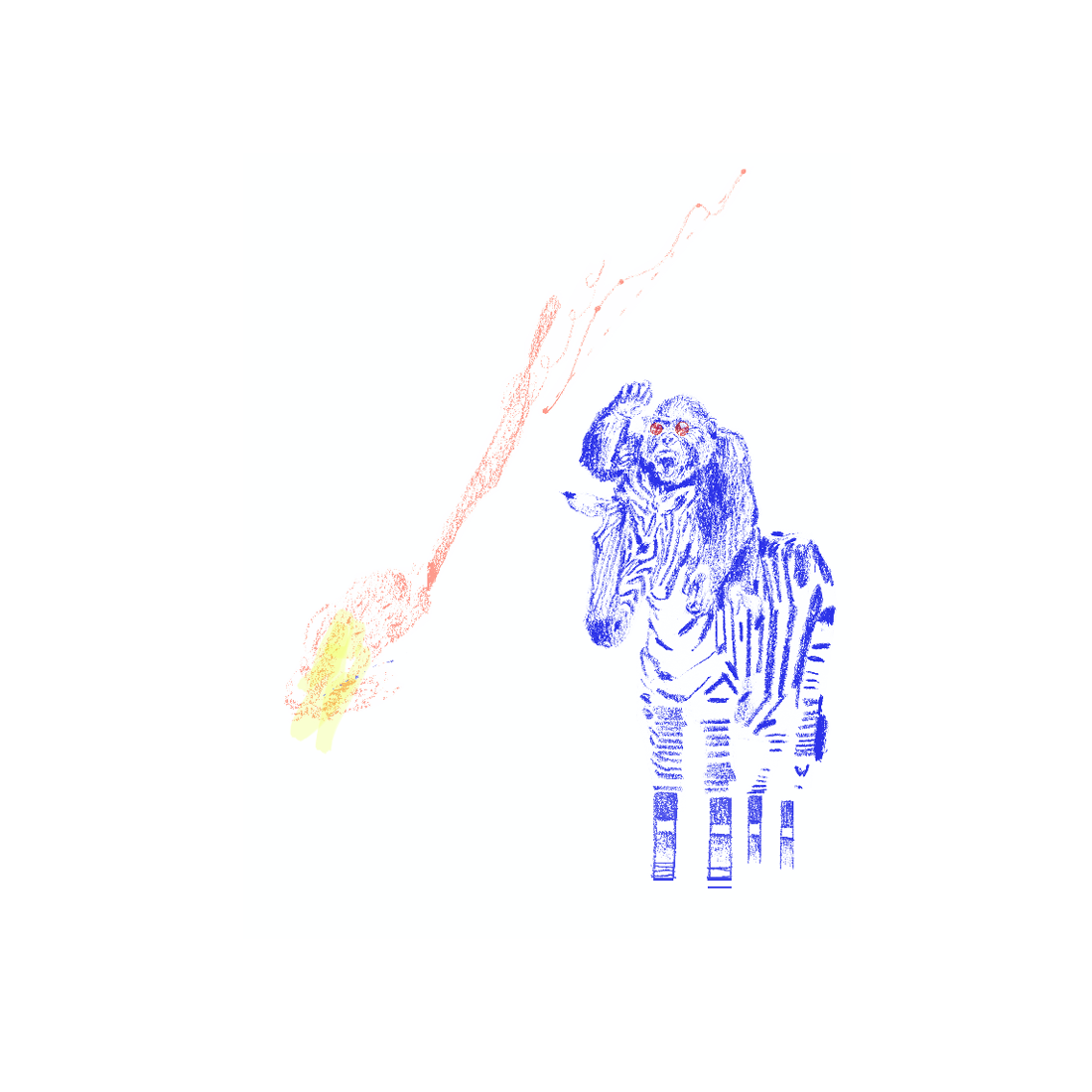
L
ife is a pain. You spend all this time learning about parametric distributions and assuming that they somehow define the world. Can’t you remember all of your statistics, economics, math, and econometrics courses? That pesky ANOVA class they made all the psychology and nursing students take? Everything was assumed to be normally distributed. What was the point of teaching that? Statistics break these assumptions all the time, and these results are more or less irrelevant to a primary care provider. Oh well, breadth requirements must be met.
Though this leads to some mathematically nice results (by nice, I mean literally the most beautiful results that I can understand), it is, in fact, lacking in its ability to predict the future. There are alternatives, we will discuss this eventually.
Though we might seek a unified law or theory of statistics, this really doesn’t help us. Here’s the problem: as soon as we have a large enough data set, we immediately start to split it into little fiefdoms that we might think are relevant to our cause. In marketing, we might split our data up into age by gender, or employment class. Suddenly our grandiose and heavy-handed statistics defining our overall population are significantly different in our subgroups. Largely, what we are interested in doing when we collect statistics is making predictions about the future or testing different responses in different groups. Think practically about this—would the average amount spent on investment be the same in the lowest decile compared to the top decile? I would argue, definitely not. Now, if I were trying to create policy or products in a relevant industry, knowing these differences in the population are terribly important.
Enter Reverend Thomas Bayes. Bayes thought about probability a bit differently. See, usually when people think about probability, they observe an experiment multiple times and report how many times it happens. For example, let’s say you wanted to see if a die was fair. For your experiment, you would roll the dice 600 times and hope to see each side of the die land face up 100 times. Maybe you would roll a two 103 times, and a four 97 times. As long as it was around 100, you would be happily convinced that the die was fair. This experiment would be boring and tedious, but we would be fairly certain the die was fair.
When Bayes started to describe probability, he thought that we should use what we already know and update our knowledge as we learn more. An example of this could not have been put more bluntly than in the aphorism coined by Dr. Theodore Woodward when he instructed his medical interns, “When you hear hoofbeats, think of horses not zebras.” Woodward’s hospital was in Maryland—where zebras don’t really roam free. Woodward’s aphorism was trying to push his interns to treat the most likely cause of their patient’s ailment. He was alluding to the fact that, oftentimes, in the presence of too much information we lose our grip on what the most obvious outcome really is. If you hear hoofbeats in Maryland, being as it is a state on the Atlantic side of the USA, it is not commonly zebra “stompin’ ground.”
This shows up in our lives in a very different way. We are shown exceptionally rare events on the news, in our social media feed, and so on. This is usually quite gripping and affects us on an emotional level. Exceptionally rare and deadly diseases or plane crashes come to mind. In our head, after being exposed to these moving pieces of information, we start to attempt to connect it in our daily lives. For instance, after listening to a Joe Rogan podcast, where he spoke of how terrible chimpanzee attacks are (they are, don’t Google it, trust me), I was honestly staring periodically at treetops in Southwark Park in London looking for any type of primate. Being as there were no primates there, I was asking myself, “If I was a primate, where would I hide?” The rabbit hole goes deep.
This is in no way restricted to negative events. Ever imagined winning the lottery? You’re just as guilty as I am. Incredibly rare events can shape our psyche in quite an unhelpful way. Pangs of fear and anxiety after a thriller or horror movie? Welcome, my friend. These emotions short circuit our better faculties.
As a card-carrying member of the human race, I don’t like to admit to being wrong or
slightly incorrect. This is especially true when analyzing my own decision-making processes. I wish all I said and did was bulletproof. But, as a card-carrying human, there’s a snowball’s chance in hell that is the case. We are guilty of thinking in a Bayesian-esque way, and also letting our emotions figure too strongly into our thought process. Sometimes, I look for chimpanzees instead of horses.
I must be satisfied with a paltry consolation prize of at least knowing when and how a thought process can go wrong. A framework I can use, when I have the time, patience, and judgement to do so, is looking at things through a Bayesian lens. This is precisely what I am trying to do as I check in for my flight to Italy tomorrow whilst
simultaneously Googling the status of the Boeing 737 Max planes. Are they still grounded? Is their software used elsewhere? I don’t know. I will think bayesian-ly and remember the odds of expiring in an aviation event is one in 5.3 million or .000000018%. I’ll take those odds.
Cameron McIntyre currently works in financial technology in the Square Mile of London. A Canadian native, Cameron graduated from McGill University with an interdisciplinary degree in mathematics and economics. Passionate about using data to make the best decision, Cameron is pursuing a masters of liberal arts at Harvard University in data science. You are likely to find Cameron on a plane exploring Europe, or assembling YouTube playlists he probably will never watch.